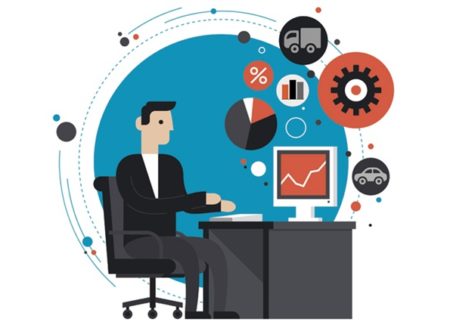
When it comes to your vehicle fleet, your company has a strategy, goals and objectives. So when it comes to analyzing how it’s doing, determine what is most important to your particular business strategy and current year objectives – and allow that to drive the metrics you will track to evaluate your fleet’s performance.
But what about the data you use to analyze your fleet? Business Intelligence tools make our jobs easier, but they are still just tools. The ability to sort through data quickly and easily makes it easier to take mental shortcuts and do something wrong, increasing the likelihood of making an analytical mistake.
Over-generalizing
Drawing conclusions on little data Small data sets run a greater risk of representing anomalies just by chance. Often in fleet, we don’t have a lot of data to answer a particular question, so be cautious about drawing quick and clear conclusions. You may think you’re looking at the average cost of cars, when you’re really looking at the average cost of Ford Fusions with around 24 months in service, driven mainly in the city of Chicago in the winter with a driver who likes to speed and leave his engine idling. Data is often very specific and literal, so overgeneralizing could easily lead to the wrong conclusions.
Ignoring or misunderstanding variance Point estimates are not always useful
A value could vary quite a bit from the average and still be within a normal and expected range. Average doesn’t tell you the full story, so reporting an expected range of values can be more informative. Case in point: Maintenance costs. Maintenance involves a lot of random chance. Just because your maintenance spend jumps from one month to another, or is higher than average, does not mean that you have a spend problem, or that this data point is actionable.
Analysis paralysis
Tracking things that are not important Remember Aesop’s fable of the fox and the cat? The fox boasts about having hundreds of escape plans, but the cat only has one. When the hunters come, the cat immediately runs up the tree, but the fox panics and can’t decide which route to use. The fox gets caught by the hounds. Analysis paralysis is much like that. Trying to track everything will not be useful. Instead, align what you track with your company’s strategic objectives.
Over-complication
Occam’s Razor Concept– Occam’s Razor states that when faced with more than one hypothesis that explains the data, choose the simpler explanation. More variables and factors and assumptions do not necessarily result in better analysis. For example, a hairy creature in the woods is more likely a bear than Bigfoot. Likewise, imagine that a driver of a bigger truck spends more on fuel. The likely reason is simply that the truck is bigger, not that the driver is stealing fuel or idling unnecessarily
Confusing correlation with causation
Let’s say, for every increase of 800 lawyers in Lagos, we increase spending on furniture by N1billion. There isn’t necessarily a clearly defined relationship between the two. It could be that another confounding variable such as increased overall wealth causes both to rise. Or, it could be random chance. There are so many variables to track that sometimes two completely unrelated variables will correlate by purely random chance. Be careful about drawing inferences when two trends move together.